Spark Mobile Growth
DT’s Ignite technology accelerates mobile growth and consumer engagement. See how DT can help you succeed:
Ready to get started?
Give your mobile growth objectives the fuel they need with Digital Turbine's solutions.
Our Partners
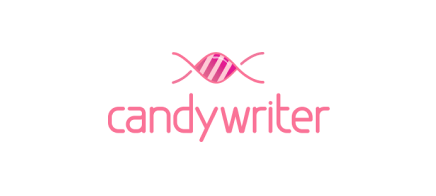

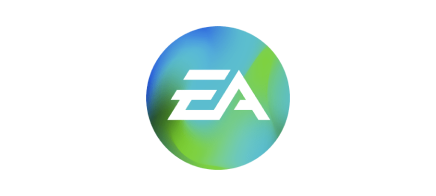
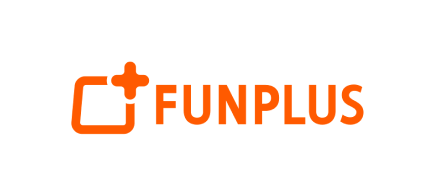
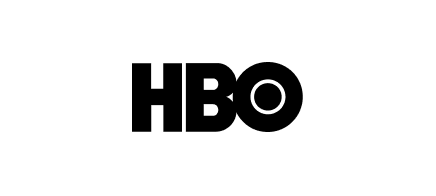
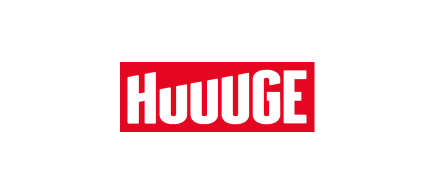
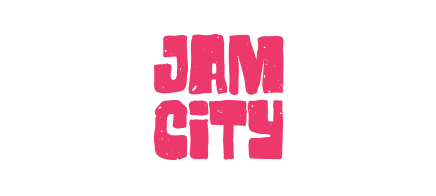
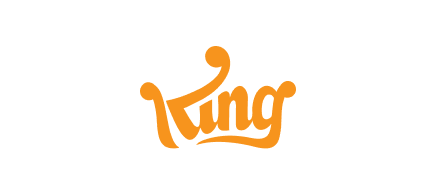
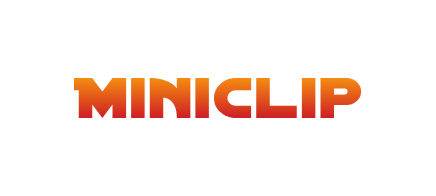
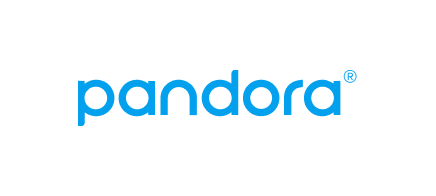
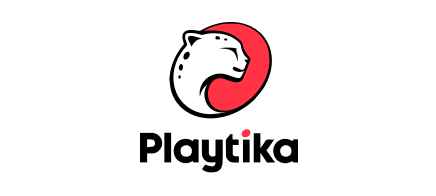
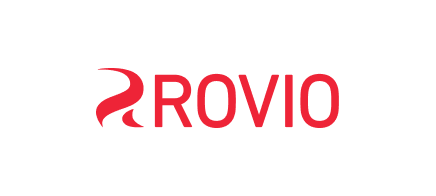

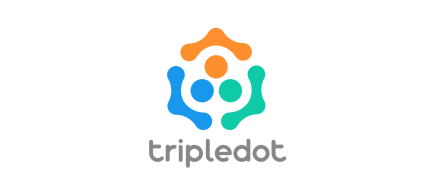
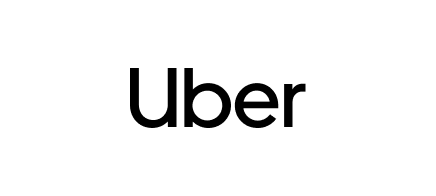

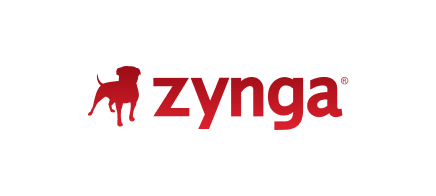
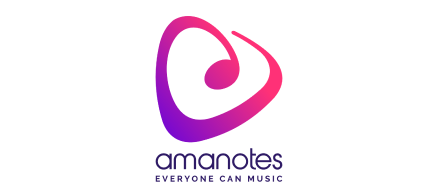
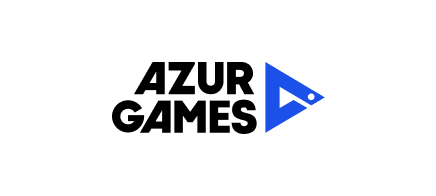
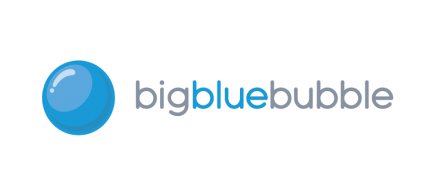
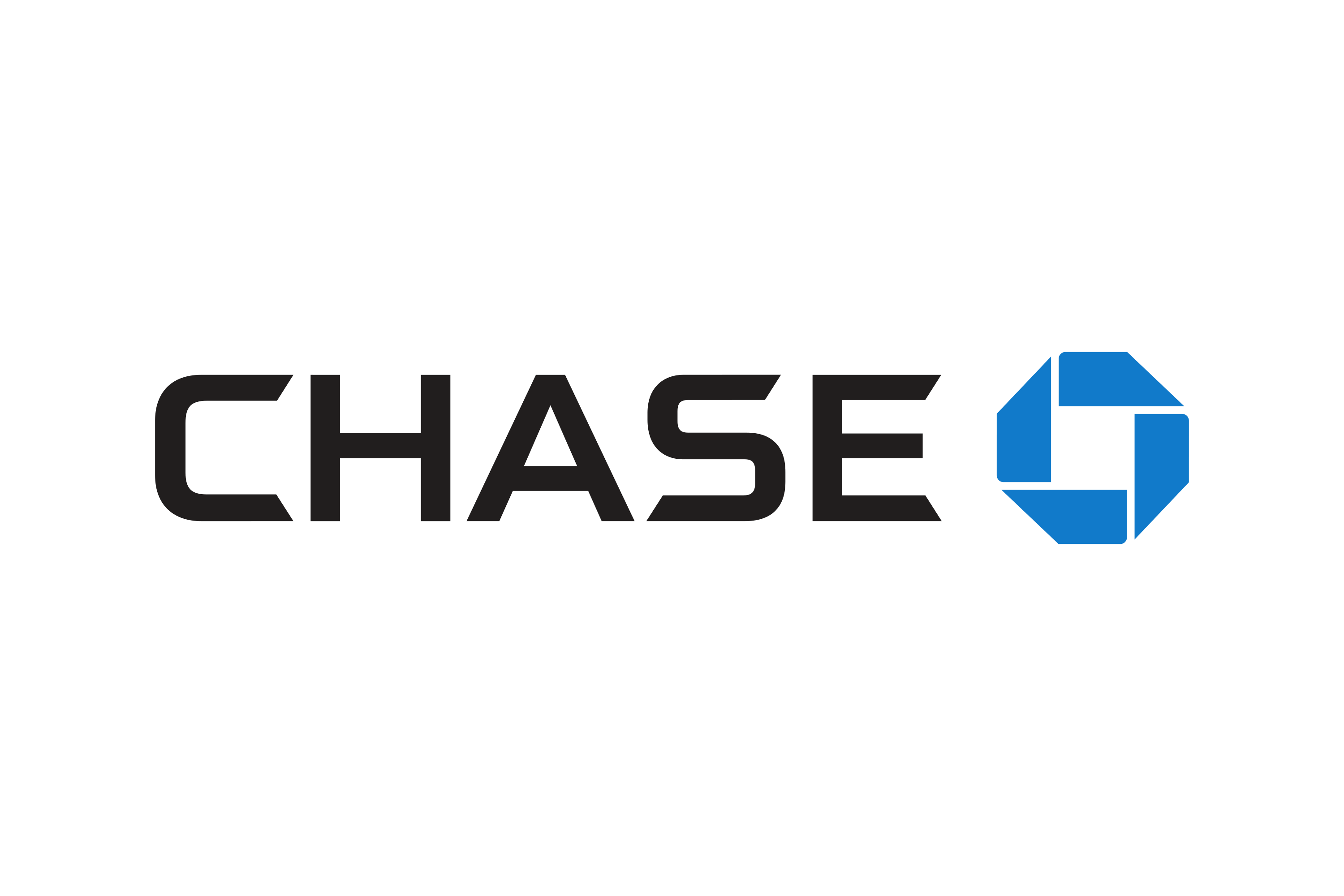
Client Success Stories
Delivering Angry Birds and Happy Users for Rovio
Digital Turbine is flying high with our ability to help deliver Rovio’s award-winning games to users around the globe. "We have been able to unlock a valuable source for acquiring new users with Digital Turbine's platform," says the Finnish game-make
Blowing Brand Awareness KPIs Away for J&J
Johnson & Johnson saw a 20% uplift in brand awareness with campaigns using DT Reach, including our Creative Labs, and Aurora video units. "We witnessed the power of mobile come to life," said the CPG and healthcare leader.
“By partnering with Digital Turbine, we’ve been able to significantly increase how we engage our subscribers with content and new apps, driving significant VAS revenue”, says the Director of VAS Programs, Top U.S. Carrier
Unlock your mobile potential
Are you ready to power your mobile success with Digital Turbine's solutions?